Abstract
Consider a Fixed Number of Clustered Areas Identified by their Geographical Coordinates that Are Monitored for the Occurrences of an Event Such as a Pandemic, Epidemic, or Migration. Data Collected on Units at All Areas Include Covariates and Environmental Factors. We Apply a Probit Transformation to the Time to Event and Embed an Isotropic Spatial Correlation Function into Our Models for Better Modeling as Compared to Existing Methodologies that Use Frailty or Copula. Composite Likelihood Technique is Employed for the Construction of a Multivariate Gaussian Random Field that Preserves the Spatial Correlation Function. the Data Are Analyzed using Counting Process and Geostatistical Formulation that Led to a Class of Weighted Pairwise Semiparametric Estimating Functions. the Estimators of Model Parameters Are Shown to Be Consistent and Asymptotically Normally Distributed under Infill-Type Asymptotic Spatial Statistics. Detailed Small Sample Numerical Studies that Are in Agreement with Theoretical Results Are Provided. the Foregoing Procedures Are Applied to the Leukemia Survival Data in Northeast England. a Comparison to Existing Methodologies Provides Improvement.
Recommended Citation
F. Z. Sainul Abdeen et al., "Statistical Modeling of Right-Censored Spatial Data using Gaussian Random Fields," Mathematics, vol. 12, no. 10, article no. 1521, MDPI, May 2024.
The definitive version is available at https://doi.org/10.3390/math12101521
Department(s)
Mathematics and Statistics
Publication Status
Open Access
International Standard Serial Number (ISSN)
2227-7390
Document Type
Article - Journal
Document Version
Final Version
File Type
text
Language(s)
English
Rights
© 2024 The Authors, All rights reserved.
Creative Commons Licensing
This work is licensed under a Creative Commons Attribution 4.0 License.
Publication Date
01 May 2024
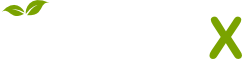
- Usage
- Downloads: 19
- Abstract Views: 5
- Captures
- Readers: 1
- Mentions
- Blog Mentions: 1
- News Mentions: 1