Knowing When to Pass: The Effect of AI Reliability in Risky Decision Contexts
Abstract
Objective: This study manipulates the presence and reliability of AI recommendations for risky decisions to measure the effect on task performance, behavioral consequences of trust, and deviation from a probability matching collaborative decision-making model. Background: Although AI decision support improves performance, people tend to underutilize AI recommendations, particularly when outcomes are uncertain. As AI reliability increases, task performance improves, largely due to higher rates of compliance (following action recommendations) and reliance (following no-action recommendations). Methods: In a between-subject design, participants were assigned to a high reliability AI, low reliability AI, or a control condition. Participants decided whether to bet that their team would win in a series of basketball games tying compensation to performance. We evaluated task performance (in accuracy and signal detection terms) and the behavioral consequences of trust (via compliance and reliance). Results: AI recommendations improved task performance, had limited impact on risk-taking behavior, and were under-valued by participants. Accuracy, sensitivity (d'), and reliance increased in the high reliability AI condition, but there was no effect on response bias (c) or compliance. Participant behavior was only consistent with a probability matching model for compliance in the low reliability condition. Conclusion: In a pay-off structure that incentivized risk-taking, the primary value of the AI recommendations was in determining when to perform no action (i.e., pass on bets). Application: In risky contexts, designers need to consider whether action or no-action recommendations will be more influential to design appropriate interventions.
Recommended Citation
H. Elder et al., "Knowing When to Pass: The Effect of AI Reliability in Risky Decision Contexts," Human Factors, SAGE Publications, Jan 2022.
The definitive version is available at https://doi.org/10.1177/00187208221100691
Department(s)
Engineering Management and Systems Engineering
Second Department
Psychological Science
Keywords and Phrases
Artificial Intelligence; Compliance; Decision-Making; Reliance; Signal Detection Theory; Trust in Automation
International Standard Serial Number (ISSN)
1547-8181; 0018-7208
Document Type
Article - Journal
Document Version
Citation
File Type
text
Language(s)
English
Rights
© 2022 SAGE Publications, All rights reserved.
Publication Date
01 Jan 2022
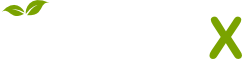
- Citations
- Citation Indexes: 7
- Usage
- Abstract Views: 10
- Captures
- Readers: 56
- Mentions
- News Mentions: 1
Comments
The author(s) disclosed receipt of the following financial support for the research, authorship, and/or publication of this article: This work was supported by DAAD PROMOS Scholarship and Division of Computer and Network Systems (grant no. 2026324).