Co-Prediction of Multiple Transportation Demands based on Deep Spatio-Temporal Neural Network
Abstract
Taxi and sharing bike bring great convenience to urban transportation. A lot of efforts have been made to improve the efficiency of taxi service or bike sharing system by predicting the next-period pick-up or drop-off demand. Different from the existing research, this paper is motivated by the following two facts: 1) From a micro view, an observed spatial demand at any time slot could be decomposed as a combination of many hidden spatial demand bases; 2) From a macro view, the multiple transportation demands are strongly correlated with each other, both spatially and temporally. Definitely, the above two views have great potential to revolutionize the existing taxi or bike demand prediction methods. Along this line, this paper provides a novel Co-prediction method based on Spatio-Temporal neural Network, namely, CoST-Net. In particular, a deep convolutional neural network is constructed to decompose a spatial demand into a combination of hidden spatial demand bases. The combination weight vector is used as a representation of the decomposed spatial demand. Then, a heterogeneous Long Short-Term Memory (LSTM) is proposed to integrate the states of multiple transportation demands, and also model the dynamics of them mixedly. Last, the environmental features such as humidity and temperature are incorporated with the achieved overall hidden states to predict the multiple demands simultaneously. Experiments have been conducted on real-world taxi and sharing bike demand data, results demonstrate the superiority of the proposed method over both classical and the state-of-the-art transportation demand prediction methods.
Recommended Citation
J. Ye et al., "Co-Prediction of Multiple Transportation Demands based on Deep Spatio-Temporal Neural Network," Proceedings of the ACM SIGKDD International Conference on Knowledge Discovery and Data Mining (2019, Anchorage, AK), pp. 305 - 313, Association for Computing Machinery (ACM), Aug 2019.
The definitive version is available at https://doi.org/10.1145/3292500.3330887
Meeting Name
25th ACM SIGKDD International Conference on Knowledge Discovery and Data Mining, KDD 2019 (2019: Aug. 4-8, Anchorage, AK)
Department(s)
Computer Science
Keywords and Phrases
Deep Neural Network; Demand Prediction; Sharing Economy; Spatio-Temporal Analysis
International Standard Book Number (ISBN)
978-145036201-6
Document Type
Article - Conference proceedings
Document Version
Citation
File Type
text
Language(s)
English
Rights
© 2019 Association for Computing Machinery (ACM), All rights reserved.
Publication Date
01 Aug 2019
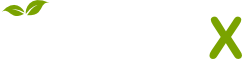
- Citations
- Citation Indexes: 133
- Patent Family Citations: 1
- Usage
- Abstract Views: 62
- Captures
- Readers: 127
Comments
This work is supported by the National Natural Science Foundation of China under Grant No. 51822802, 51778033, U1811463, and 71531001, the Science and Technology Major Project of Beijing under Grant No. Z171100005117001.