Iteratively Training Classifiers for Circulating Tumor Cell Detection
Abstract
The number of Circulating Tumor Cells (CTCs) in blood provides an indication of disease progression and tumor response to chemotherapeutic agents. Hence, routine detection and enumeration of CTCs in clinical blood samples have significant applications in early cancer diagnosis and treatment monitoring. In this paper, we investigate two classifiers for image-based CTC detection: (1) Support Vector Machine (SVM) with hard-coded Histograms of Oriented Gradients (HoG) features; and (2) Convolutional Neural Network (CNN) with automatically learned features. For both classifiers, we present an effective and efficient training algorithm, by which the most representative negative samples are iteratively collected to accurately define the classification boundary between positive and negative samples. The two iteratively trained classifiers are validated on a challenging dataset with high performance.
Recommended Citation
Y. Mao et al., "Iteratively Training Classifiers for Circulating Tumor Cell Detection," Proceedings of the International Symposium on Biomedical Imaging (2015, Brooklyn, NY), pp. 190 - 194, Institute of Electrical and Electronics Engineers (IEEE), Jul 2015.
The definitive version is available at https://doi.org/10.1109/ISBI.2015.7163847
Meeting Name
2015 IEEE 12th International Symposium on Biomedical Imaging (ISBI) (2015: Apr. 16-19 Brooklyn, NYA)
Department(s)
Computer Science
Keywords and Phrases
Blood; Cells; Classification (of Information); Convolution; Diagnosis; Iterative Methods; Medical Imaging; Neural Networks; Support Vector Machines; Tumors; Chemotherapeutic Agents; Circulating Tumor Cells; Classification Boundary; Convolutional Neural Network; Disease Progression; Histograms of Oriented Gradients (Hog); Training Algorithms; Treatment Monitoring; Image Classification; Iterative Training; Support Vector Machine
International Standard Book Number (ISBN)
978-1479923748
International Standard Serial Number (ISSN)
1945-7928
Document Type
Article - Conference proceedings
Document Version
Citation
File Type
text
Language(s)
English
Rights
© 2015 Institute of Electrical and Electronics Engineers (IEEE), All rights reserved.
Publication Date
01 Jul 2015
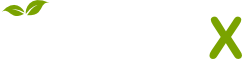
- Citations
- Citation Indexes: 21
- Patent Family Citations: 3
- Usage
- Abstract Views: 7
- Captures
- Readers: 19