Human Activity Recognition using Wearable Sensors by Deep Convolutional Neural Networks
Abstract
Human physical activity recognition based on wearable sen-sors has applications relevant to our daily life such as health-care. How to achieve high recognition accuracy with low computational cost is an important issue in the ubiquitous computing. Rather than exploring handcrafted features from time-series sensor signals, we assemble signal sequences of accelerometers and gyroscopes into a novel activity image, which enables Deep Convolutional Neural Networks (DCNN) to automatically learn the optimal features from the activ-ity image for the activity recognition task. Our proposed approach is evaluated on three public datasets and it out-performs state-of-The-Arts in terms of recognition accuracy and computational cost.
Recommended Citation
W. Jiang and Z. Yin, "Human Activity Recognition using Wearable Sensors by Deep Convolutional Neural Networks," Proceedings of the 2015 ACM Multimedia Conference MM'15 (2017, Brisbane, Australia), pp. 1307 - 1310, Association for Computing Machinery (ACM), Oct 2015.
The definitive version is available at https://doi.org/10.1145/2733373.2806333
Meeting Name
2015 ACM Multimedia Conference MM'15 (2017: Oct. 26-30, Brisbane, Australia)
Department(s)
Computer Science
Keywords and Phrases
Convolution; Image Recognition; Neural Networks; Pattern Recognition; Ubiquitous Computing; Wearable Technology; Activity Recognition; Computational Costs; Convolutional Neural Network; Human Activity Recognition; Physical Activity; Recognition Accuracy; State of the Art; Wearable Computing; Wearable Sensors; activity Image; Deep Convolutional Neural Networks; Wearable Computing
International Standard Book Number (ISBN)
978-1450334594
Document Type
Article - Conference proceedings
Document Version
Citation
File Type
text
Language(s)
English
Rights
© 2015 Association for Computing Machinery (ACM), All rights reserved.
Publication Date
01 Oct 2015
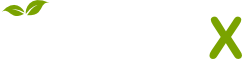
- Citations
- Citation Indexes: 554
- Patent Family Citations: 2
- Usage
- Abstract Views: 176
- Captures
- Readers: 483