Abstract
The availability of Electronic Health Records (EHR) in health care settings provides terrific opportunities for early detection of patients' potential diseases. While many data mining tools have been adopted for EHR-based disease early detection, Linear Discriminant Analysis (LDA) is one of the most widely used statistical prediction methods. To improve the performance of LDA for early detection of diseases, we proposed to leverage CRDA - Covariance-Regularized LDA classifiers on top of diagnosis-frequency vector data representation. Specifically, CRDA employs a sparse precision matrix estimator derived based on graphical lasso to boost the accuracy of LDA classifiers. Algorithm analysis demonstrates that the error bound of graphical lasso estimator can intuitively lower the misclassification rate of LDA models. We performed extensive evaluation of CRDA using a large-scale real-world EHR dataset - CHSN for predicting mental health disorders (e.g., depression and anxiety) in college students from 10 US universities. We compared CRDA with other regularized LDA and downstream classifiers. The result shows CRDA outperforms all baselines by achieving significantly higher accuracy and F1 scores.
Recommended Citation
J. Bian et al., "Early Detection of Diseases using Electronic Health Records Data and Covariance-regularized Linear Discriminant Analysis," 2017 IEEE EMBS International Conference on Biomedical and Health Informatics, BHI 2017, pp. 457 - 460, article no. 7897304, Institute of Electrical and Electronics Engineers, Apr 2017.
The definitive version is available at https://doi.org/10.1109/BHI.2017.7897304
Department(s)
Computer Science
International Standard Book Number (ISBN)
978-150904179-4
Document Type
Article - Conference proceedings
Document Version
Citation
File Type
text
Language(s)
English
Rights
© 2024 Institute of Electrical and Electronics Engineers, All rights reserved.
Publication Date
11 Apr 2017
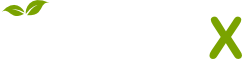
- Citations
- Citation Indexes: 10
- Usage
- Downloads: 6
- Captures
- Readers: 37
- Mentions
- News Mentions: 1