Abstract
A top-down progressive deepening method is developed for efficient mining of multiple-level association rules from large transaction databases based on the Apriori principle. A group of variant algorithms is proposed based on the ways of sharing intermediate results, with the relative performance tested and analyzed. The enforcement of different interestingness measurements to find more interesting rules, and the relaxation of rule conditions for finding `level-crossing' association rules, are also investigated in the paper. Our study shows that efficient algorithms can be developed from large databases for the discovery of interesting and strong multiple-level association rules.
Recommended Citation
J. Han and Y. Fu, "Mining Multiple-level Association Rules in Large Databases," IEEE Transactions on Knowledge and Data Engineering, vol. 11, no. 5, pp. 798 - 805, Institute of Electrical and Electronics Engineers, Dec 1999.
The definitive version is available at https://doi.org/10.1109/69.806937
Department(s)
Computer Science
International Standard Serial Number (ISSN)
1041-4347
Document Type
Article - Journal
Document Version
Citation
File Type
text
Language(s)
English
Rights
© 2024 Institute of Electrical and Electronics Engineers, All rights reserved.
Publication Date
01 Dec 1999
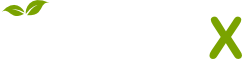
- Citations
- Citation Indexes: 293
- Patent Family Citations: 2
- Usage
- Downloads: 6
- Abstract Views: 1
- Captures
- Readers: 90
Comments
Natural Sciences and Engineering Research Council of Canada, Grant NSERC-A3723