Abstract
With the enhanced usage of Artificial Intelligence (AI) driven applications, the researchers often face challenges in improving the accuracy of the data classification models, while trading off the complexity. In this paper, we address the classification of time series data using the Long Short-Term Memory (LSTM) network while focusing on the activation functions. While the existing activation functions such as sigmoid and tanh are used as LSTM internal activations, the customizability of these activations stays limited. This motivates us to propose a new family of activation functions, called log-sigmoid, inside the LSTM cell for time series data classification, and analyze its properties. We also present the use of a linear transformation (e.g., log tanh) of the proposed log-sigmoid activation as a replacement of the traditional tanh function in the LSTM cell. Both the cell activation as well as recurrent activation functions inside the LSTM cell are modified with log-sigmoid activation family while tuning the log bases. Further, we report a comparative performance analysis of the LSTM model using the proposed and the state-of-the-art activation functions on multiple public time-series databases.
Recommended Citation
S. Das, "Sigmoid Activation-Based Long Short-Term Memory for Time Series Data Classification," IEEE Transactions on Artificial Intelligence, Institute of Electrical and Electronics Engineers, Jan 2023.
The definitive version is available at https://doi.org/10.1109/TAI.2023.3265641
Department(s)
Computer Science
Document Type
Article - Journal
Document Version
Citation
File Type
text
Language(s)
English
Rights
© 2023 Institute of Electrical and Electronics Engineers, All rights reserved.
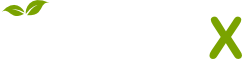
- Citations
- Citation Indexes: 5
- Usage
- Downloads: 396
- Abstract Views: 12
- Captures
- Readers: 11
- Mentions
- Blog Mentions: 1