Rapid Airfoil Design Optimization Via Neural Networks-Based Parameterization and Surrogate Modeling
Abstract
Aerodynamic optimization based on computational fluid dynamics (CFD) is a powerful design approach because it significantly reduces the design time compared with the human manual design. However, CFD-based optimization can still take hours to converge because it requires repeatedly running computationally expensive flow simulations. To further shorten the design optimization time, we propose a fast, interactive design framework that allows us to complete an airfoil aerodynamic optimization within a few seconds. This framework is made efficient through a B-spline-based generative adversarial network model for shape parameterization, which filters out unrealistic airfoils for a reduced design space that contains all relevant airfoil shapes. Moreover, we use a combination of multilayer perceptron, recurrent neural networks, and mixture of experts for surrogate modeling to enable both scalar (drag and lift) and vector (pressure distribution) response predictions for a wide range of Mach numbers (0.3 to 0.7) and Reynolds numbers (104 to 1010). To verify our proposed framework, we compare the optimization results with the ones computed by direct CFD-based optimization for subsonic and transonic conditions. The results show that the optimal designs and the aerodynamic quantities (lift, drag, and pressure distribution) obtained by our proposed framework agree well with the ones computed by direct CFD-based optimizations and evaluations. The proposed framework is being integrated into a web-based interactive aerodynamic design framework that allows users to predict drag, lift, moment, pressure distribution, and optimal airfoil shapes for a wide range of flow conditions within seconds.
Recommended Citation
X. Du et al., "Rapid Airfoil Design Optimization Via Neural Networks-Based Parameterization and Surrogate Modeling," Aerospace Science and Technology, vol. 113, article no. 106701, Elsevier; Elsevier Masson, Jun 2021.
The definitive version is available at https://doi.org/10.1016/j.ast.2021.106701
Department(s)
Mechanical and Aerospace Engineering
International Standard Serial Number (ISSN)
1270-9638
Document Type
Article - Journal
Document Version
Citation
File Type
text
Language(s)
English
Rights
© 2023 Elsevier; Elsevier Masson, All rights reserved.
Publication Date
01 Jun 2021
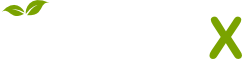
- Citations
- Policy Citations: 1
- Citation Indexes: 127
- Usage
- Abstract Views: 4
- Captures
- Readers: 90
- Mentions
- News Mentions: 1
Comments
Air Force Office of Scientific Research, Grant FA9550-15-1-0038