Li-Ion Battery State of Health Estimation based on an Improved Single Particle Model
Abstract
Health-conscious battery management is one of the main facilitators for widespread commercialization of Li-ion batteries as the primary power source in electrified transportation and portable electronics and as the backup source in stationary energy storage systems. The majority of the existing Battery Management Systems (BMSs) define battery State of Health (SOH) in terms of internal resistance increase or battery capacity decay and use various open-loop criteria based on the battery cycle number and/or operating conditions to determine its SOH. However, considering the wide range of operating conditions and current profiles for Li-ion batteries, the use of a closed-loop SOH estimation approach based on the measureable quantities of the battery along with a battery model is of great importance. In this work, the battery internal resistance increase which can be attributed to various chemical and mechanical degradation mechanisms is considered as the measure of the battery SOH. In order to estimate the SOH, a modified reduced-order electrochemical model based on the Single Particle (SP) Li-ion battery model is proposed to improve the traditional SP model accuracy. This model not only incorporates an analytical expression for the electrolyte-phase potential difference, it is also capable of accurately predicting the battery performance over a wide range of operating currents by considering the effects of the unmodeled dynamics. Finally, this model integrated with an adaptive output-injection observer to estimate the SP model states and the output model uncertainties, can be used to estimate the internal resistance increase during the battery lifetime. The modeling and estimation results are validated via a comparison to the full-order electrochemical model simulations.
Recommended Citation
N. Lotfi et al., "Li-Ion Battery State of Health Estimation based on an Improved Single Particle Model," Proceedings of the American Control Conference (2017, Seattle, WA), pp. 86 - 91, Institute of Electrical and Electronics Engineers (IEEE), May 2017.
The definitive version is available at https://doi.org/10.23919/ACC.2017.7962935
Meeting Name
2017 American Control Conference, ACC 2017 (2017: May 24-26, Seattle, WA)
Department(s)
Mechanical and Aerospace Engineering
Research Center/Lab(s)
Intelligent Systems Center
Second Research Center/Lab
Center for High Performance Computing Research
Keywords and Phrases
Adaptive observer; Battery State of Health (SOH) estimation; Electrochemical model-based state estimation; Improved Single Particle (SP) battery model; Li-ion battery
International Standard Book Number (ISBN)
978-150905992-8
International Standard Serial Number (ISSN)
0743-1619
Document Type
Article - Conference proceedings
Document Version
Citation
File Type
text
Language(s)
English
Rights
© 2017 American Automatic Control Council (AACC), All rights reserved.
Publication Date
01 May 2017
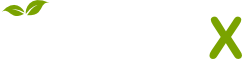
- Citations
- Citation Indexes: 48
- Patent Family Citations: 1
- Usage
- Abstract Views: 6
- Captures
- Readers: 61