Efficient Mini-Batch Stochastic Gradient Descent with Centroidal Voronoi Tessellation for Pde-Constrained Optimization under Uncertainty
Abstract
The study of optimal control problems under uncertainty plays an important role in scientific numerical simulations. This class of optimization problems is frequently utilized in engineering, biology and finance. Although stochastic gradient descent is a well-known stochastic optimization technique for solving the approximate optimal control problem, it often exhibits a slow convergence rate due to the inherent variance in gradient approximation. To address this issue, we propose a more efficient stochastic optimization algorithm based on Centroidal Voronoi Tessellation sampling. This strategy reduces the error in gradient estimation compared to the conventional mini-batch stochastic gradient descent method. Our approach involves dividing the whole snapshot set into several Voronoi cells with low variance and extracting samples with good uniformity from each region to construct an unbiased estimation of the full gradient. Our method can economically and stably approximate numerical optimal control functions even with a fixed step size. Numerical results demonstrate that the proposed method is a reliable algorithm with great potential for applications in the field of optimization.
Recommended Citation
L. Chen et al., "Efficient Mini-Batch Stochastic Gradient Descent with Centroidal Voronoi Tessellation for Pde-Constrained Optimization under Uncertainty," Physica D: Nonlinear Phenomena, vol. 467, article no. 134216, Elsevier, Nov 2024.
The definitive version is available at https://doi.org/10.1016/j.physd.2024.134216
Department(s)
Mathematics and Statistics
Keywords and Phrases
Centroidal Voronoi Tessellation; Mini-batch stochastic gradient descent; Optimization with uncertainty; PDE-constrained optimization; Sampling methods
International Standard Serial Number (ISSN)
0167-2789
Document Type
Article - Journal
Document Version
Citation
File Type
text
Language(s)
English
Rights
© 2024 Elsevier, All rights reserved.
Publication Date
01 Nov 2024
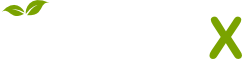
- Citations
- Citation Indexes: 1
- Usage
- Abstract Views: 3
- Captures
- Readers: 1
- Mentions
- News Mentions: 1