Methodology for Long-Term Prediction of Time Series
Abstract
In This Paper, a Global Methodology for the Long-Term Prediction of Time Series is Proposed. This Methodology Combines Direct Prediction Strategy and Sophisticated Input Selection Criteria: K-Nearest Neighbors Approximation Method (K-Nn), Mutual Information (Mi) and Nonparametric Noise Estimation (Nne). a Global Input Selection Strategy that Combines Forward Selection, Backward Elimination (Or Pruning) and Forward-Backward Selection is Introduced. This Methodology is Used to Optimize the Three Input Selection Criteria (K-Nn, Mi and Nne). the Methodology is Successfully Applied to a Real Life Benchmark: The Poland Electricity Load Dataset. © 2007 Elsevier B.v. All Rights Reserved.
Recommended Citation
A. Sorjamaa et al., "Methodology for Long-Term Prediction of Time Series," Neurocomputing, vol. 70, no. 16 thru 18, pp. 2861 - 2869, Elsevier, Oct 2007.
The definitive version is available at https://doi.org/10.1016/j.neucom.2006.06.015
Department(s)
Engineering Management and Systems Engineering
Keywords and Phrases
Direct prediction; Input selection; k-Nearest neighbors; Least squares support vector machines; Mutual information; Nonparametric noise estimation; Recursive prediction; Time series prediction
International Standard Serial Number (ISSN)
0925-2312
Document Type
Article - Journal
Document Version
Citation
File Type
text
Language(s)
English
Rights
© 2024 Elsevier, All rights reserved.
Publication Date
01 Oct 2007
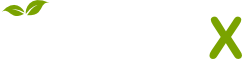
- Citations
- Citation Indexes: 353
- Patent Family Citations: 1
- Policy Citations: 2
- Usage
- Abstract Views: 1
- Captures
- Readers: 311