Abstract
In the Community of Computational Materials Science, One of the Challenges in Hierarchical Multiscale Modeling is Information-Passing from One Scale to Another, especially from the Molecular Model to the Continuum Model. a Machine-Learning-Enhanced Approach, Proposed in This Paper, Provides an Alternative Solution. in the Developed Hierarchical Multiscale Method, Molecular Dynamics Simulations in the Molecular Model Are Conducted First to Generate a Dataset, Which Represents Physical Phenomena at the Nanoscale. the Dataset is Then Used to Train a Material Failure/defect Classification Model and Stress Regression Models. Finally, the Well-Trained Models Are Implemented in the Continuum Model to Study the Mechanical Behaviors of Materials at the Macroscale. Multiscale Modeling and Simulation of a Molecule Chain and an Aluminum Crystalline Solid Are Presented as the Applications of the Proposed Method. in Addition to Support Vector Machines, Extreme Learning Machines with Single-Layer Neural Networks Are Employed Due to their Computational Efficiency.
Recommended Citation
S. Xiao et al., "A Machine-Learning-Enhanced Hierarchical Multiscale Method for Bridging from Molecular Dynamics to Continua," Neural Computing and Applications, vol. 32, no. 18, pp. 14359 - 14373, Springer, Sep 2020.
The definitive version is available at https://doi.org/10.1007/s00521-019-04480-7
Department(s)
Engineering Management and Systems Engineering
Keywords and Phrases
Continuum model; Extreme learning machine; Hierarchical multiscale method; Molecular model
International Standard Serial Number (ISSN)
1433-3058; 0941-0643
Document Type
Article - Journal
Document Version
Citation
File Type
text
Language(s)
English
Rights
© 2024 Springer, All rights reserved.
Publication Date
01 Sep 2020
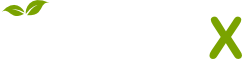
- Citations
- Patent Family Citations: 1
- Citation Indexes: 18
- Usage
- Downloads: 30
- Abstract Views: 1
- Captures
- Readers: 72