Abstract
Three networks are compared for low false alarm stock trend predictions. Short-term trends, particularly attractive for neural network analysis, can be used profitably in scenarios such as option trading, but only with significant risk. Therefore, we focus on limiting false alarms, which improves the risk/reward ratio by preventing losses. To predict stock trends, we exploit time delay, recurrent, and probabilistic neural networks (TDNN, RNN, and PNN, respectively), utilizing conjugate gradient and multistream extended Kalman filter training for TDNN and RNN. We also discuss different predictability analysis techniques and perform an analysis of predictability based on a history of daily closing price. Our results indicate that all the networks are feasible, the primary preference being one of convenience
Recommended Citation
D. C. Wunsch et al., "Comparative Study of Stock Trend Prediction using Time Delay, Recurrent and Probabilistic Neural Networks," IEEE Transactions on Neural Networks, Institute of Electrical and Electronics Engineers (IEEE), Jan 1998.
The definitive version is available at https://doi.org/10.1109/72.728395
Department(s)
Electrical and Computer Engineering
Second Department
Computer Science
Keywords and Phrases
Kalman Filters; Conjugate Gradient Methods; Conjugate Gradient Training; Daily Closing Price; Feedforward Neural Nets; Filtering Theory; Forecasting Theory; Learning (Artificial Intelligence); Low False Alarm; Multilayer Perceptrons; Multistream Extended Kalman Filter Training; Nonlinear Filters; Option Trading; Predictability Analysis Techniques; Probabilistic Neural Networks; Recurrent Neural Nets; Recurrent Neural Networks; Risk/Reward Ratio; Short-Term Trends; Stock Markets; Stock Trend Prediction; Time Delay Neural Networks; Time Series
International Standard Serial Number (ISSN)
1045-9227
Document Type
Article - Journal
Document Version
Final Version
File Type
text
Language(s)
English
Rights
© 1998 Institute of Electrical and Electronics Engineers (IEEE), All rights reserved.
Publication Date
01 Jan 1998
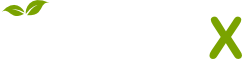
- Citations
- Citation Indexes: 402
- Usage
- Downloads: 2271
- Abstract Views: 17
- Captures
- Readers: 320
- Mentions
- References: 1