Abstract
The increasing penetration of inverter-based resources (IBRs) calls for an advanced active and reactive power (PQ) control strategy in microgrids. To enhance the controllability and flexibility of the IBRs, this paper proposed an adaptive PQ control method with trajectory tracking capability, combining model-based analysis, physics-informed reinforcement learning (RL), and power hardware-in-the-loop (HIL) experiments. First, model-based analysis proves that there exists an adaptive proportional-integral controller with time-varying gains that can ensure any exponential PQ output trajectory of IBRs. These gains consist of a constant factor and an exponentially decaying factor, which are then obtained using a model-free deep reinforcement learning approach known as the twin delayed deeper deterministic policy gradient. With the model-based derivation, the learning space of the RL agent is narrowed down from a function space to a real space, which reduces the training complexity significantly. Finally, the proposed method is verified through numerical simulation in MATLAB-Simulink and power HIL experiments in the CURENT center.With the physics-informed learning method, exponential response time constants can be freely assigned to IBRs, and they can follow any predefined trajectory without complicated gain tuning.
Recommended Citation
B. She et al., "Inverter PQ Control With Trajectory Tracking Capability For Microgrids Based On Physics-informed Reinforcement Learning," IEEE Transactions on Smart Grid, Institute of Electrical and Electronics Engineers, Jan 2023.
The definitive version is available at https://doi.org/10.1109/TSG.2023.3277330
Department(s)
Electrical and Computer Engineering
Publication Status
Early Access
Keywords and Phrases
Adaptation models; Adaptive systems; inverter PQ control; inverter-based resources; Inverters; Microgrids; Microgrids; physics-informed reinforcement learning; PI control; power hardware-in-the-loop experiment; Trajectory; trajectory tracking; Transfer functions
International Standard Serial Number (ISSN)
1949-3061; 1949-3053
Document Type
Article - Journal
Document Version
Citation
File Type
text
Language(s)
English
Rights
© 2023 Institute of Electrical and Electronics Engineers, All rights reserved.
Publication Date
01 Jan 2023
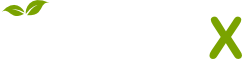
- Citations
- Citation Indexes: 16
- Usage
- Downloads: 403
- Abstract Views: 7
- Captures
- Readers: 19
- Mentions
- News Mentions: 1