Abstract
This article presents a novel efficient experience-replay-based adaptive dynamic programming (ADP) for the optimal control problem of a class of nonlinear dynamical systems within the Hamiltonian-driven framework. The quasi-Hamiltonian is presented for the policy evaluation problem with an admissible policy. With the quasi-Hamiltonian, a novel composite critic learning mechanism is developed to combine the instantaneous data with the historical data. In addition, the pseudo-Hamiltonian is defined to deal with the performance optimization problem. Based on the pseudo-Hamiltonian, the conventional Hamilton–Jacobi–Bellman (HJB) equation can be represented in a filtered form, which can be implemented online. Theoretical analysis is investigated in terms of the convergence of the adaptive critic design and the stability of the closed-loop systems, where parameter convergence can be achieved under a weakened excitation condition. Simulation studies are investigated to verify the efficacy of the presented design scheme.
Recommended Citation
Y. Yang et al., "Hamiltonian-Driven Adaptive Dynamic Programming with Efficient Experience Replay," IEEE Transactions on Neural Networks and Learning Systems, Institute of Electrical and Electronics Engineers, Jan 2022.
The definitive version is available at https://doi.org/10.1109/TNNLS.2022.3213566
Department(s)
Electrical and Computer Engineering
Keywords and Phrases
Convergence; Dynamic programming; Hamiltonian-driven adaptive dynamic programming (ADP); Hamilton–Jacobi–Bellman (HJB) equation; Iterative algorithms; Learning systems; Mathematical models; Optimal control; Optimization; pseudo-Hamiltonian; quasi-Hamiltonian; relaxed excitation condition
International Standard Serial Number (ISSN)
2162-2388; 2162-237X
Document Type
Article - Journal
Document Version
Final Version
File Type
text
Language(s)
English
Rights
© 2023 Institute of Electrical and Electronics Engineers, All rights reserved.
Publication Date
01 Jan 2022
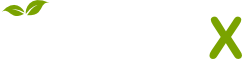
- Citations
- Citation Indexes: 115
- Usage
- Downloads: 202
- Abstract Views: 1
- Captures
- Readers: 10
- Mentions
- Blog Mentions: 1
- News Mentions: 1