Transformer Fault Diagnosis using Deep Neural Network
Abstract
Analysis of dissolved gases in transformer oil is one of the practical methods for identifying the different types of faults in oil-insulated power transformers. Dissolved gas analysis (DGA) is often exercised as part of the maintenance process, and the Duval Triangle is a commonly applied method for classifying transformer faults. This paper proposes using the deep neural network to identify transformer fault type. Due to limited availability of field data, we simulate DGA data samples along with the fault type determined by Duval Triangle. Numerical results show that the deep neutral network provides very high accuracy in fault type identification and outperforms other learning methods such as k-nearest neighbor (k-NN) algorithm and random forest classifier method.
Recommended Citation
H. Mehdipourpicha et al., "Transformer Fault Diagnosis using Deep Neural Network," Proceedings of the 2019 IEEE PES Innovative Smart Grid Technologies Asia (2019, Chengdu, China), pp. 4241 - 4245, Institute of Electrical and Electronics Engineers (IEEE), May 2019.
The definitive version is available at https://doi.org/10.1109/ISGT-Asia.2019.8881052
Meeting Name
2019 IEEE PES Innovative Smart Grid Technologies Asia, ISGT 2019 (2019: May 21-24, Chengdu, China)
Department(s)
Electrical and Computer Engineering
Keywords and Phrases
Deep Neural Network; Dissolved Gas Analysis; Duval Triangle; Transformer Fault Diagnosis
International Standard Book Number (ISBN)
978-172813520-5
Document Type
Article - Conference proceedings
Document Version
Citation
File Type
text
Language(s)
English
Rights
© 2019 Institute of Electrical and Electronics Engineers (IEEE), All rights reserved.
Publication Date
01 May 2019
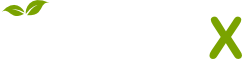
- Citations
- Citation Indexes: 23
- Policy Citations: 1
- Usage
- Abstract Views: 17
- Captures
- Readers: 22