Abstract
The high performance of FPGA (Field Programmable Gate Array) in image processing applications is justified by its flexible reconfigurability, its inherent parallel nature and the availability of a large amount of internal memories. Lately, the Stochastic Computing (SC) paradigm has been found to be significantly advantageous in certain application domains including image processing because of its lower hardware complexity and power consumption. However, its viability is deemed to be limited due to its serial bitstream processing and excessive run-time requirement for convergence. To address these issues, a novel approach is proposed in this work where an energy-efficient implementation of SC is accomplished by introducing fast-converging Quasi-Stochastic Number Generators (QSNGs) and parallel stochastic bitstream processing, which are well suited to leverage FPGA's reconfigurability and abundant internal memory resources. The proposed approach has been tested on the Virtex-4 FPGA, and results have been compared with the serial and parallel implementations of conventional stochastic computation using the well-known SC edge detection and multiplication circuits. Results prove that by using this approach, execution time, as well as the power consumption are decreased by a factor of 3.5 and 4.5 for the edge detection circuit and multiplication circuit, respectively.
Recommended Citation
R. Seva et al., "Energy-Efficient FPGA-Based Parallel Quasi-Stochastic Computing," Journal of Low Power Electronics and Applications, vol. 7, no. 4, MDPI AG, Nov 2017.
The definitive version is available at https://doi.org/10.3390/jlpea7040029
Department(s)
Electrical and Computer Engineering
Keywords and Phrases
Edge Detection; FPGA; Quasi-Stochastic Number Generator; Reconfigurability; Stochastic Computing
International Standard Serial Number (ISSN)
2079-9268
Document Type
Article - Journal
Document Version
Final Version
File Type
text
Language(s)
English
Rights
© 2017 MDPI AG, All rights reserved.
Creative Commons Licensing
This work is licensed under a Creative Commons Attribution 4.0 License.
Publication Date
01 Nov 2017
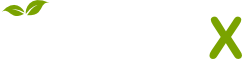
- Citations
- Citation Indexes: 8
- Usage
- Downloads: 520
- Abstract Views: 4
- Captures
- Readers: 12
- Mentions
- Blog Mentions: 1