Abstract
Predicting solar irradiance has been an important topic in renewable energy generation. Prediction improves the planning and operation of photovoltaic systems and yields many economic advantages for electric utilities. The irradiance can be predicted using statistical methods such as artificial neural networks (ANN), support vector machines (SVM), or autoregressive moving average (ARMA). However, they either lack accuracy because they cannot capture long-term dependency or cannot be used with big data because of the scalability. This paper presents a method to predict the solar irradiance using deep neural networks. Deep recurrent neural networks (DRNNs) add complexity to the model without specifying what form the variation should take and allow the extraction of high-level features. The DRNN is used to predict the irradiance. The data utilized in this study is real data obtained from natural resources in Canada. The simulation of this method will be compared to several common methods such as support vector regression and feedforward neural networks (FNN). The results show that deep learning neural networks can outperform all other methods, as the performance tests indicate.
Recommended Citation
A. Alzahrani et al., "Solar Irradiance Forecasting using Deep Neural Networks," Procedia Computer Science, vol. 114, pp. 304 - 313, Elsevier, Nov 2017.
The definitive version is available at https://doi.org/10.1016/j.procs.2017.09.045
Meeting Name
Complex Adaptive Systems Conference: Engineering Cyber Physical Systems (2017: Oct. 30-Nov. 1, Chicago, IL)
Department(s)
Electrical and Computer Engineering
Second Department
Engineering Management and Systems Engineering
Research Center/Lab(s)
Intelligent Systems Center
Keywords and Phrases
Adaptive Systems; Big Data; Complex Networks; Electric Utilities; Embedded Systems; Feedforward Neural Networks; Forecasting; Neural Networks; Photovoltaic Cells; Recurrent Neural Networks; Renewable Energy Resources; Solar Radiation; Support Vector Machines; Autoregressive Moving Average; DRNN; Irradiance; Learning Neural Networks; Power; Renewable Energy Generation; Solar; Support Vector Regression (SVR); Deep Neural Networks; DNN; PV
International Standard Serial Number (ISSN)
1877-0509
Document Type
Article - Conference proceedings
Document Version
Final Version
File Type
text
Language(s)
English
Rights
© 2017 Elsevier, All rights reserved.
Creative Commons Licensing
This work is licensed under a Creative Commons Attribution-Noncommercial-No Derivative Works 4.0 License.
Publication Date
01 Nov 2017
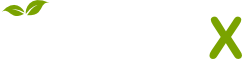
- Citations
- Citation Indexes: 226
- Usage
- Downloads: 354
- Abstract Views: 12
- Captures
- Readers: 414
- Mentions
- References: 2
Included in
Electrical and Computer Engineering Commons, Operations Research, Systems Engineering and Industrial Engineering Commons