Recurrent Neural Network Based Prediction of Epileptic Seizures in Intra- and Extracranial EEG
Abstract
Predicting the onset of epileptic seizure is an important and difficult biomedical problem, which has attracted substantial attention of the intelligent computing community over the past two decades. We apply recurrent neural networks (RNN) combined with signal wavelet decomposition to the problem. We input raw EEG and its wavelet-decomposed subbands into RNN training/testing, as opposed to specific signal features extracted from EEG. To the best of our knowledge this approach has never been attempted before. The data used included both scalp and intracranial EEG recordings obtained from two epileptic patients. We demonstrate that the existence of a 'preictal' stage (immediately preceding seizure) of some minutes duration is quite feasible.
Predicting the onset of epileptic seizure is an important and difficult biomedical problem, which has attracted substantial attention of the intelligent computing community over the past two decades. We apply recurrent neural networks (RNN) combined with signal wavelet decomposition to the problem. We input raw EEG and its wavelet-decomposed subbands into RNN training/testing, as opposed to specific signal features extracted from EEG. To the best of our knowledge this approach has never been attempted before. The data used included both scalp and intracranial EEG recordings obtained from two epileptic patients. We demonstrate that the existence of a 'preictal' stage (immediately preceding seizure) of some minutes duration is quite feasible.
Recommended Citation
A. Petrosian et al., "Recurrent Neural Network Based Prediction of Epileptic Seizures in Intra- and Extracranial EEG," Neurocomputing, vol. 30, pp. 201 - 218, Elsevier, Jan 2000.
The definitive version is available at https://doi.org/10.1016/S0925-2312(99)00126-5
Department(s)
Electrical and Computer Engineering
International Standard Serial Number (ISSN)
0925-2312
Document Type
Article - Journal
Document Version
Citation
File Type
text
Language(s)
English
Rights
© 2000 Elsevier, All rights reserved.
Publication Date
01 Jan 2000
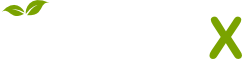
- Citations
- Citation Indexes: 236
- Patent Family Citations: 1
- Usage
- Abstract Views: 29
- Captures
- Readers: 155
- Mentions
- References: 2