Lung Segmentation in CT Images using a Fully Convolutional Neural Network with Multi-Instance and Conditional Adversary Loss
Abstract
Lung segmentation is usually the first step of lung CT image analysis and plays an important role in lung disease diagnosis. We propose an efficient end-to-end fully convolutional neural network to segment lungs with different diseases in CT images. We introduce a multi-instance loss and a conditional adversary loss to the neural network in order to solve the segmentation problem for more severe pathological conditions. Our method is capable of solving the lung segmentation problem under normal, moderate and severe pathological conditions, which is validated on 3 public benchmark datasets with different diseases.
Recommended Citation
T. Zhao et al., "Lung Segmentation in CT Images using a Fully Convolutional Neural Network with Multi-Instance and Conditional Adversary Loss," Proceedings of the IEEE International Symposium on Biomedical Imaging (2018, Washington, DC), Institute of Electrical and Electronics Engineers (IEEE), Apr 2018.
The definitive version is available at https://doi.org/10.1109/ISBI.2018.8363626
Meeting Name
IEEE 15th Annual International Symposium on Biomedical Imaging, ISBI 2018 (2018: Apr. 4-7, Washington, DC)
Department(s)
Computer Science
Research Center/Lab(s)
Intelligent Systems Center
Keywords and Phrases
Lung segmentation; CT; Fully convolutional neural network; Multi-instance; Conditional adversarial network
International Standard Book Number (ISBN)
978-1-5386-3636-7
International Standard Serial Number (ISSN)
1945-8452
Document Type
Article - Conference proceedings
Document Version
Citation
File Type
text
Language(s)
English
Rights
© 2018 Institute of Electrical and Electronics Engineers (IEEE), All rights reserved.
Publication Date
07 Apr 2018
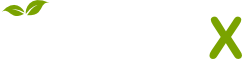
- Citations
- Citation Indexes: 30
- Patent Family Citations: 1
- Usage
- Abstract Views: 27
- Captures
- Readers: 24