Abstract
Wood-frame structures are used in almost 90% of residential buildings in the United States. It is thus imperative to rapidly and accurately assess the damage of wood-frame structures in the wake of an earthquake event. This study aims to develop a machine-learning-based seismic classifier for a portfolio of 6,113 wood-frame structures near the New Madrid Seismic Zone (NMSZ) in which synthesized ground motions are adopted to characterize potential earthquakes. This seismic classifier, based on a multilayer perceptron (MLP), is compared with existing fragility curves developed for the same wood-frame buildings near the NMSZ. This comparative study indicates that the MLP seismic classifier and fragility curves perform equally well when predicting minor damage. However, the MLP classifier is more accurate than the fragility curves in prediction of moderate and severe damage. Compared with the existing fragility curves with earthquake intensity measures as inputs, machine-learning-based seismic classifiers can incorporate multiple parameters of earthquakes and structures as input features, thus providing a promising tool for accurate seismic damage assessment in a portfolio scale. Once trained, the MLP classifier can predict damage classes of the 6,113 structures within 0.07 s on a general-purpose computer.
Recommended Citation
X. Yuan et al., "Machine Learning-Based Seismic Damage Assessment Of Residential Buildings Considering Multiple Earthquake And Structure Uncertainties," Natural Hazards Review, vol. 24, no. 3, article no. 04023024, American Society of Civil Engineers, Aug 2023.
The definitive version is available at https://doi.org/10.1061/NHREFO.NHENG-1681
Department(s)
Civil, Architectural and Environmental Engineering
Second Department
Engineering Management and Systems Engineering
Publication Status
Early Access
International Standard Serial Number (ISSN)
1527-6996; 1527-6988
Document Type
Article - Journal
Document Version
Citation
File Type
text
Language(s)
English
Rights
© 2023 American Society of Civil Engineers, All rights reserved.
Publication Date
01 Aug 2023
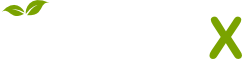
- Citations
- Citation Indexes: 3
- Usage
- Downloads: 301
- Abstract Views: 22
- Captures
- Readers: 20
- Mentions
- News Mentions: 1
Included in
Architectural Engineering Commons, Operations Research, Systems Engineering and Industrial Engineering Commons, Structural Engineering Commons
Comments
U.S. Department of Transportation, Grant 00059709