Evaluation and Prediction of the Hazard Potential Level of Dam Infrastructures Using Computational Artificial Intelligence Algorithms
Alternative Title
Evaluation and Prediction of the Hazard Potential Level of Dams Using Artificial Intelligence Algorithms
Abstract
Failures of dams cause immense property and environmental damages and take thousands of lives. As such, the goal of this paper is to evaluate and predict the hazard potential level of dams in the US using a comparative approach based on computational artificial intelligence (AI) algorithms. The research methodology comprised data collection from the National Inventory of Dams (NID); data preprocessing; data processing; and model selection and evaluation. To this end, the authors: (1) identified the best subset of variables that affect the prediction of the hazard potential level of dams in the US; (2) investigated the performance of two AI computational algorithms: artificial neural networks (ANNs) and k-nearest neighbors (KNNs) for the evaluation and prediction of the hazard potential levels of US dams; and (3) developed a decision support tool that could be used by the agencies responsible for the management of dams in the US with the capability to predict the hazard potential with good accuracy. The obtained results reflected that the ANN algorithm yielded better accuracy compared to the KNN algorithm. In addition, the conclusions indicated that 19 variables pertaining to dams in the US could affect the hazard potential level of dams. The output is a decision support system that is able to evaluate the hazard potential of dams with a prediction accuracy of 85.70%. This study contributes to the management in engineering’s body of knowledge by devising a data-driven framework that is valuable for dams’ owners and authorities. Ultimately, the developed computational AI algorithm could be used to evaluate and predict the hazard potential level of US dams with good accuracy while minimizing the efforts, time, and costs associated with formal inspection of the dams.
Recommended Citation
R. Assaad and I. H. El-adaway, "Evaluation and Prediction of the Hazard Potential Level of Dam Infrastructures Using Computational Artificial Intelligence Algorithms," Journal of Management in Engineering, vol. 36, no. 5, American Society of Civil Engineers (ASCE), Sep 2020.
The definitive version is available at https://doi.org/10.1061/(ASCE)ME.1943-5479.0000810
Department(s)
Civil, Architectural and Environmental Engineering
Document Type
Article - Journal
Document Version
Citation
File Type
text
Language(s)
English
Rights
© 2020 American Society of Civil Engineers (ASCE), All rights reserved.
Publication Date
01 Sep 2020
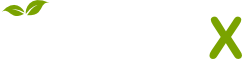
- Citations
- Citation Indexes: 30
- Policy Citations: 1
- Usage
- Abstract Views: 3
- Captures
- Readers: 65