Fusing and Refining Convolutional Neural Network Models for Assembly Action Recognition in Smart Manufacturing
Abstract
Assembly carries paramount importance in manufacturing. Being able to support workers in real time to maximize their positive contributions to assembly is a tremendous interest of manufacturers. Human action recognition has been a way to automatically analyze and understand worker actions to support real-time assistance for workers and facilitate worker–machine collaboration. Assembly actions are distinct from activities that have been well studied in the action recognition literature. Actions taken by assembly workers are intricate, variable, and may involve very fine motions. Therefore, recognizing assembly actions remains a challenging task. This paper proposes to simply use only two wearable devices that respectively capture the inertial measurement unit data of each hand of workers. Then, two convolutional neural network models with an identical architecture are independently trained using the two sources of inertial measurement unit data to respectively recognize the right-hand and the left-hand actions of an assembly worker. Classification results of the two convolutional neural network models are fused to yield a final action recognition result because the two hands often collaborate in assembling operations. Transfer learning is implemented to adapt the action recognition models to subjects whose data have not been included in dataset for training the models. One operation in assembling a Bukito three-dimensional printer, which is composed of seven actions, is used to demonstrate the implementation and assessment of the proposed method. Results from the study have demonstrated that the proposed approach effectively improves the prediction accuracy at both the action level and the subject level. Work of the paper builds a foundation for building advanced action recognition systems such as multimodal sensor-based action recognition.
Recommended Citation
M. Al-Amin et al., "Fusing and Refining Convolutional Neural Network Models for Assembly Action Recognition in Smart Manufacturing," Proceedings of the Institution of Mechanical Engineers, Part C: Journal of Mechanical Engineering Science, vol. 236, no. 4, pp. 2046 - 2059, SAGE Publications, Feb 2022.
The definitive version is available at https://doi.org/10.1177/0954406220931547
Department(s)
Engineering Management and Systems Engineering
Second Department
Mechanical and Aerospace Engineering
Keywords and Phrases
action recognition; inertial measurement unit sensor; Manufacturing assembly; model fusion; transfer learning
International Standard Serial Number (ISSN)
2041-2983; 0954-4062
Document Type
Article - Journal
Document Version
Citation
File Type
text
Language(s)
English
Rights
© 2023 SAGE Publications, All rights reserved.
Publication Date
01 Feb 2022
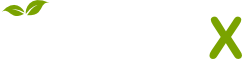
- Citations
- Citation Indexes: 24
- Patent Family Citations: 1
- Usage
- Abstract Views: 33
- Captures
- Readers: 35
- Mentions
- News Mentions: 1
Comments
National Science Foundation, Grant 1954548